Discover How Generative AI is Shaping Society and Revolutionizing Technology
2023/10/30
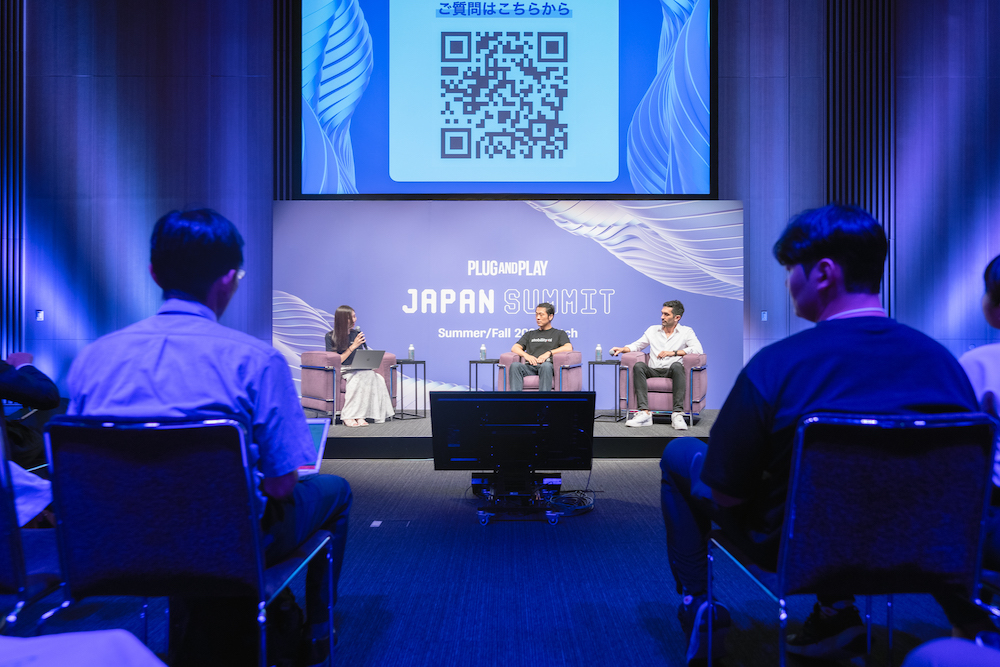
During our Japan Summit Summer/Fall 2023, the visionary founders of two cutting-edge startups, Recursive INC and Stability AI Japan K.K., delved deep into the profound impact of AI’s evolution. They explored its effect on the harmonious coexistence of humans and AI, innovative business models, future prospects, and the role of technology in shaping society to come.
(This article is based on the panel discussion themed “How Generative AI Would Impact Society” at Japan Summit Summer/Fall 2023 on September 15, 2023)
Jerry Chi
Stability AI Japan K.K. Head of Japan
Jerry is a Taiwanese-American fluent in Chinese, Japanese, Korean, and English. He is a 2006 Stanford University School of Engineering graduate and a 2012 Wharton School of the University of Pennsylvania graduate. He launched Stability AI's Japan office in January 2023. He has previously held analytics and machine learning-related positions at Google, Supercell, Smart News, and Indeed. His passion is the application of generative AI and machine learning to the creative field. (photo on the left)
Tiago Ramalho
Recursive INC Co-founder and CEO
Tiago attained a Master's degree in Theoretical/Mathematical Physics and a Ph.D. in Biophysics from Ludwig-Maximilians-University Munich. After graduation, he joined Google DeepMind. As a senior research engineer, he worked on cutting-edge projects in reinforcement learning, predictive modeling, and self-managed learning, publishing numerous papers in international journals such as Nature. He then joined an AI startup, Cogent Labs, as a lead research scientist and came to Japan. In August 2020, he co-founded Recursive Inc. and became its president. (photo on the right)
Risa Hamada
Ventures Analyst, Plug and Play Japan
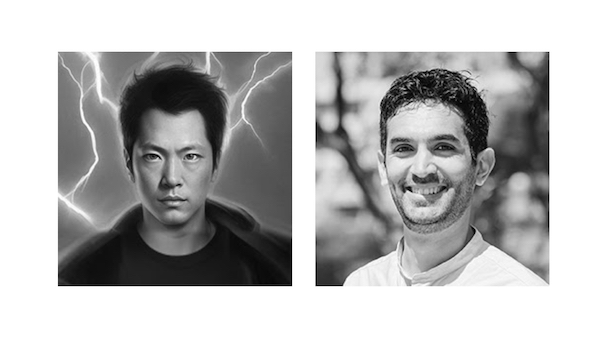
How generative AI differs from the previous AI models
--Generative AI has been one of the hottest topics this year. How do you see the excitement and impact that generative AI is bringing into our society?
- Jerry:
Technology is improving and expanding very rapidly. With the release of Stable Diffusion, our image generation AI, last year, there was rapid adoption. The open-source community developed a lot of variations of it, and it was built into various tools and apps.
Previously, AI was mostly for predicting the number or classifying spam. However, generative AI can create new things and help humans think of ideas. It also can create documents, images, sounds, or movies in ways that you couldn’t with previous AI. It has a really big impact on society in terms of productivity and creativity.
--How do you see the excitement and the impact on the business side?
- Tiago:
Generative AI has transformed how we are talking to businesses. In the past, the executives would laugh at us when we told them we needed to collect thousands of data sets for projects. But now, with generative AI, these tasks can be done in a zero-shot manner. Zero-shot means you don’t need to train the model on that specific data set.
Now, if you give us access to your data, we get a model in and do some prompt engineering, which is much easier than training a model from scratch. Then, we are going to get some pipelines and extract the data from unstructured documents, which is the thing usually preventing the adoption of automation in enterprises. A lot of the data is made to be read and consumed by humans, who don’t write data in the way that computers do, like JSON.
So, with these large language models, we can do this conversion from unstructured data to structured data. It increased the adoption of automation, which made things very interesting and opened up a lot of opportunities that just weren’t there before.
Opportunities for startups in the generative AI field
--From the successful generative AI startup’s point of view, where do you think the chances for growth underlie for startups who are going into this field?
- Jerry:
I think there are a ton of opportunities now. For most startups, it’s tough to spend a lot of money on foundation model training like we are doing, so a lot of startups are building apps and services on top of the foundational models that are available. Some of them are available through API, and some of them are available for download, which you can put on your own server.
There is a big gap between what these models can do and actual adoption by companies and governments. Just by making a model easier to use or providing an easy-to-understand interface, you can massively drive the value and adoption above what the original model could do.
--There is a lot of competition in the application layer. When it comes to actually building the applications, how do you think startups can differentiate the solution to stand out?
- Jerry:
One way is speed. For Japan, just looking at what’s going on in the US and then trying to put that over to Japan would be a simple but potentially effective strategy.
Another way is getting access to specific data through business development. For example, our company made a deal with a company called AudioSparx, which provides audio such as music for things like movies and ads. We were able to build a music generation model based on that data, which now we negotiated for the exclusive right to build a generative AI on that data. If your company has its own IP or is able to customize models to that data, it can also be a moat for competition.
- Tiago:
This is flashing technology, but at the end of the day, it’s all about generating business value. Finding a niche where you can deliver value is going to help you drum up everything. For small companies they should build on top of models that are stable and commercially realized and try to solve a problem that enterprises are facing in a very specific niche.
Clients have a lot of concerns about data leakage. That is one problem with API models like OpenAI. Even with all the assurances, sometimes clients are not willing to send their data to a server overseas. For those customers, we have to rely on open-source solutions.
The other issue is training the workforce. The people that are doing things that can be automated by AI need to be reskilled. A lot of organizations try to reskill the people they already have to use these new technologies. I think there is a potential market opportunity for startups that are going to help humans use it.
How to choose the best generative AI models
--What do you think people should look at when choosing which LLM they are to utilize?
- Jerry:
You want to consider the balance between your requirements, the cost, and the speed. Each LLM has different costs per token and different speeds to influence. Open models can be used on your own server, and closed models can’t, which might be a problem. Also, some models are biased to certain uses. Some people use ChatGPT for everything. But actually, they can use another model, which is much smaller and faster, to achieve similar results from their specific use case.
- Tiago:
I agree with Jerry. The price of GPT-4 can seem reasonable, but when you pick up an actual use case and multiply it, it can be a significant cost. You can have a much smaller model that weighs fewer parameters and is cheaper to influence.
Not only enterprises but a lot of people are worrying about AI security. I think having open-source models is one of the ways to have stability, because if everyone has access to open sources, then everyone can start developing and training their own fine-tuned models that are aligned with their values. Humans have various sets of values, so why don’t we have multiple AIs that have different sets of values and then come to a compromise? From a game theory perspective, that seems like a better, more stable outcome, rather than having open AI take place entirely.
When does singularity happen?
--When is singularity coming into your view? Is this happening in 20 years, or are we almost there?
- Tiago:
We have already had several AI winters and AI summers where, at some point, it seems like progress is going very fast, and other times it seems like progress is very slow. But this is normal. When there is a new technology, we have a breakthrough, and then progress happens very fast because people build a lot on it, but then it slows down. That is just normal scientific progress.
Right now, we are on one of those exponential curves where LLM grows a lot. But is it going to sustain itself, or is it going to be more like previous AI cycles? It’s hard to predict when a specific event is going to happen because we don’t know how long the plateau will last, and we don’t know when this new thing will unlock another cycle of exponent.
- Jerry:
If I have to estimate the specific time, I would say around 2030. But if you asked me that question two years ago, I would have said 2045. A lot of experts had to adjust their predictions in the last two years. Can we achieve singularity with the current paradigm? By training larger and larger models based on transformer architectures, just like scaling up the existing approach, or do we need a completely different approach, like spiking neural networks?
There is a non-negligible chance that with the current paradigm, we can achieve the singularity because there are already a lot of signs of intelligence in AI models being able to do things that they were never trained on. By combining different AI models in interesting ways, it’s like producing outputs that you would not think would be possible. It’s fascinating.
Big tech vs startups
--Who do you think is going to win this field, big tech or startups?
- Jerry:
I think big tech companies will do well. However, I also think there are a lot of things that startups can do that big enterprises cannot. Startups can take big risks, but some big tech companies were risk-aversive. That is how some of them fell behind OpenAI. Also, some companies don’t want to partner with big tech, because they directly compete with them or they are afraid that big tech will take their idea. Instead, they might choose to work with a startup that is more neutral, flexible, fast, and customizable to their needs. I think a lot of startups can find their niche and find their unique value.
- Tiago:
The economy is huge, and the big players are very well-positioned to win the infrastructure game. Because, they can procure a number of chips, or they can even design their own chips.
When it comes to infrastructure, it’s likely that the big players will continue their stronghold on the market. But when it comes to the application layer focusing on issues and solving problems, startups are much more able to maneuver. Even in the Gold Rush, there were people selling picks and shovels, but then there were the people actually finding the gold. I think the startups are the ones that find the gold.
Will people lose their jobs due to these technological advancements?
--Do you think people will be losing jobs due to this technological advancement?
- Jerry:
Some people are already losing jobs. Japan is relatively uninfluenced because, legally and culturally, it’s more difficult to lay off people than most other countries. With any kind of new technology, there will be changes in the workforce. But the question is, are there other new jobs coming out that have the potential to be more interesting? I believe there are a ton of new jobs around people who can leverage AI or even indirectly benefit from AI to do something more creative or build better apps, better games, and better services, so I am very excited to see new jobs and changes in the structure of the workforce.
- Tiago:
You need to type something into AI for it to give you an answer. Humans are the ones to drive things forward, to push them to come up with creative solutions, understand the situation, and figure out how to move things forward. So I think humans will be needed more than ever actually. We are going to have to push everyone into the human side of communication and high-level problem-solving strategy.
Utilization of generative AI
--How are you going to accelerate the usage of generative AI in different industries?
- Jerry:
We are working hard on making more varied and better models. Many people might know us just for Stable Diffusion, our image generation model. Actually, we also make language models in English and Japanese. We also released an image-to-text model for Japanese and a music and sound-generating model (Stable Audio) as well. I think there are a lot of applications in the use cases in marketing, games, design, and beyond. We might be targeting too many industries, but I’m just excited about a lot of these opportunities.
- Tiago:
We are looking more at how we use this model to solve complete business problems. A lot of our work is talking to people in the field who sometimes don’t even use software, let alone AI, and trying to drag them into the real world. There are very complicated and deep problems preventing the adoption of these technologies relating to workflow, so bringing a new generative AI will help bring more of those new cases into automation. Demonstrating that those problems can be solved with AI, I think it’s going to help more and more companies start with all these technologies.